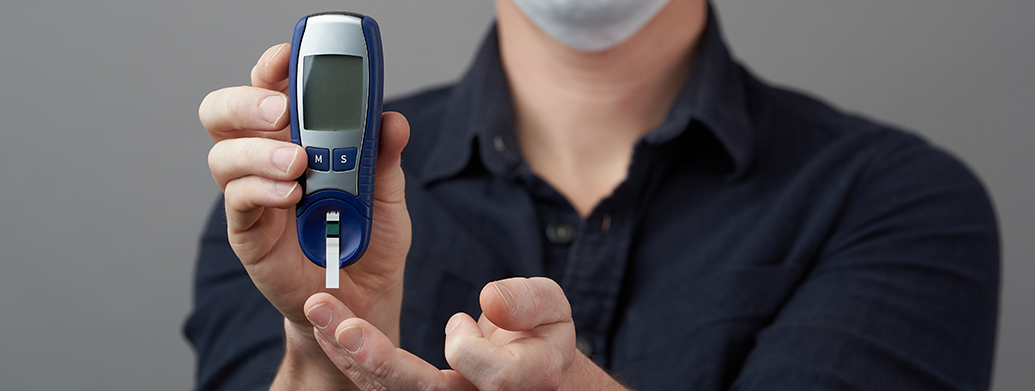
Glooko users show a slight trend toward better glucose control during the pandemic.
People with diabetes face a greater chance of experiencing serious complications caused by the coronavirus. To understand the impact of COVID-19 on people with diabetes, we analyzed glucose, insulin, and activity trends from users of Glooko solutions in the United States. We have measured data before and after mid-March, when the COVID-19 pandemic was declared as a national emergency and most state-level shelter-in-place orders came into effect.
Our main takeaways from the data analysis are that after shelter-in-place started, weekends and weekdays blend together when it comes to blood glucose levels. We also see a slight trend toward better glucose control and slightly lower and more consistent carb intake. There is however no change in insulin amounts.
To support people with diabetes and healthcare providers during the pandemic, we are offering a secure, privacy-protected remote-care solution at no charge to enable safe, connected patient care. This solution allows individuals to access ongoing medical care with their healthcare providers by facilitating remote appointments. People with diabetes are also able to track their glucose, insulin, and lifestyle data such as food and activity right in the Glooko Mobile app.
KEY TAKEAWAYS
Weekends and weekdays blend together
Prior to COVID-19-related shelter-in-place orders across the United States (around mid-March), we observed a weekly pattern consisting of relatively higher average blood glucose on the weekends, with the peak happening on Sundays. Similarly, time in range (TIR) for CGM users tended to be highest during the week and lowest on Sundays. Starting in early to mid-March, average glucose values decreased and the daily fluctuations over the week became less pronounced (Fig. 1, 2). Average glucose and TIR on weekends eventually resembled weekday levels pre-COVID-19 (Fig. 1, 2, 3).
The data show that since the national shelter-in-place order was implemented in the US in mid-March, the day-to-day fluctuations in glycemia that previously showed a weekly pattern began to dissolve, presumably as the day-to-day lifestyle and behavior on weekdays and weekends became increasingly similar.
Note: the slight peak in average glucose on April 12-13 corresponds to Easter Sunday and Monday.
Fig 1.
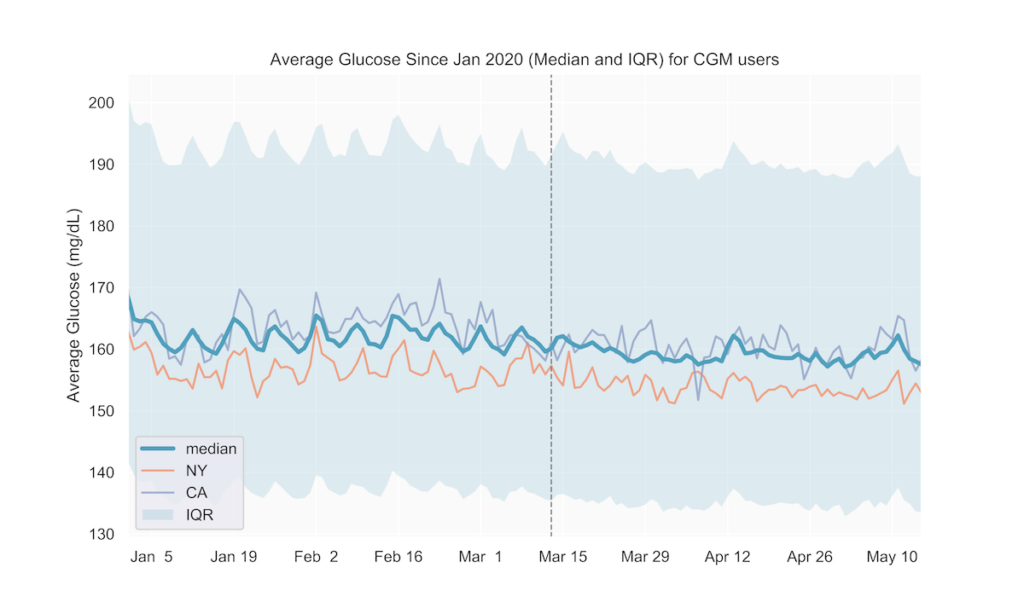
Fig 2.
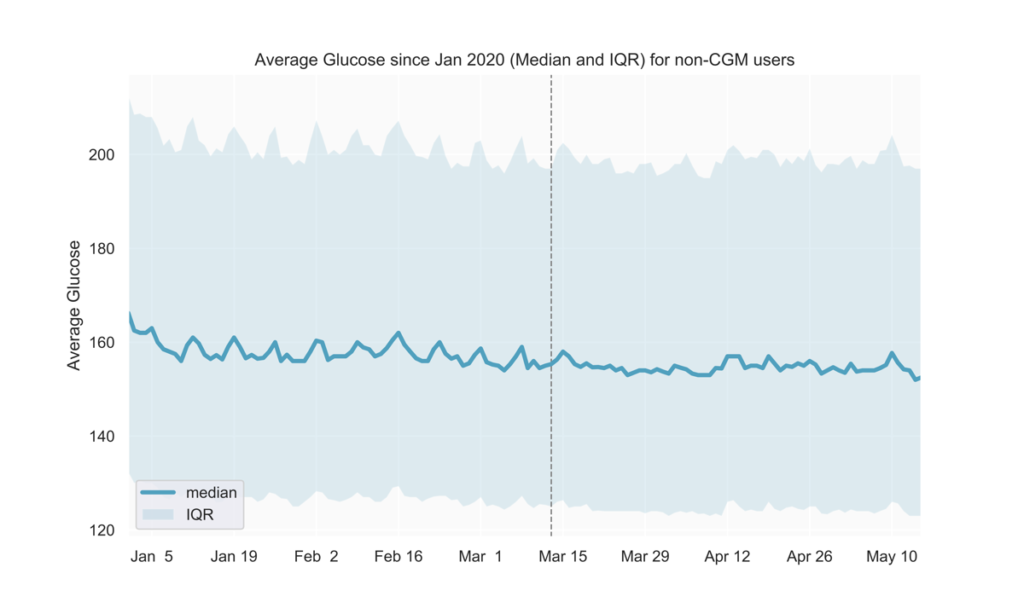
Fig 3.
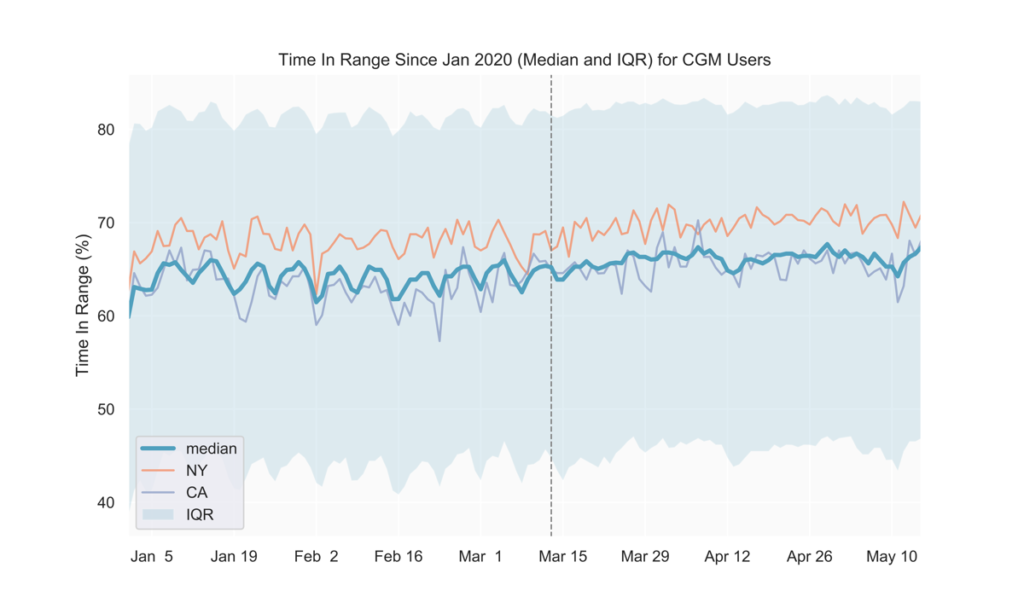
Slight trend toward better glucose control
After mid-March, we observed lower average blood glucose levels and hyperglycemia rates, along with slightly higher TIR (Fig. 1-4). These could possibly result from staying at home leading to people with diabetes having more time for diabetes management.
Fig 4.
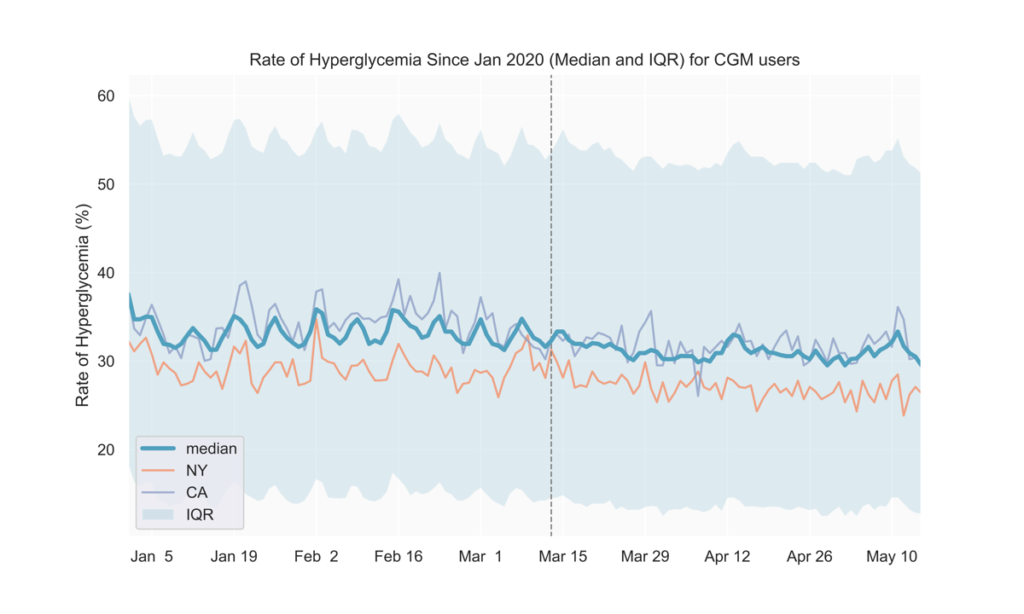
See the tables below for comparisons of glucose outcomes before and after March 13 (when the Federal emergency was announced). The differences in TIR, average glucose, and rate of hyperglycemia were statistically significant (p<0.0001).
CGM users |
Pre March 13
(3-week period) |
Post March 13
(3-week period) |
TIR mean (IQR) |
62.3% (49.3, 76.5) |
63.3% (49.8, 78.2) |
Average Glucose mean (IQR) |
166.4 mg/dL (142.8, 186.4) |
164.6 mg/dL (140.2, 185.3) |
Rate of Hyperglycemia mean (IQR) |
35.3% (19.8, 49.3) |
34.3% (18.1, 48.6) |
Non-CGM users |
Pre March 13 (3-week period) |
Post March 13 (3-week period) |
Average Glucose mean (IQR) |
169.2 mg/dL (132.1, 195.4) |
167.5 mg/dL (129.5, 194.1) |
States with early adoption of shelter-in-place directives align with national trends
We also analyzed the above metrics at the state level to find out if shelter-in-place impacts on lifestyle and glycemic management in individual states affect our users’ glucose differently than the national average. As seen in Fig. 1 and 3, states adopting shelter-in-place relatively earlier (e.g., NY, CA) do not seem to show deviations from the national trends thus far. We will continue to track individual states as they gradually reopen to detect if any new trends emerge in the next few weeks.
Slightly more consistent carb intake
Pre-COVID-19, carb intake was generally lower during the week and higher on weekends. Among people with diabetes who are insulin pump users, we observed less pronounced daily fluctuations over the week following mid-March (Fig. 5).
Fig 5.
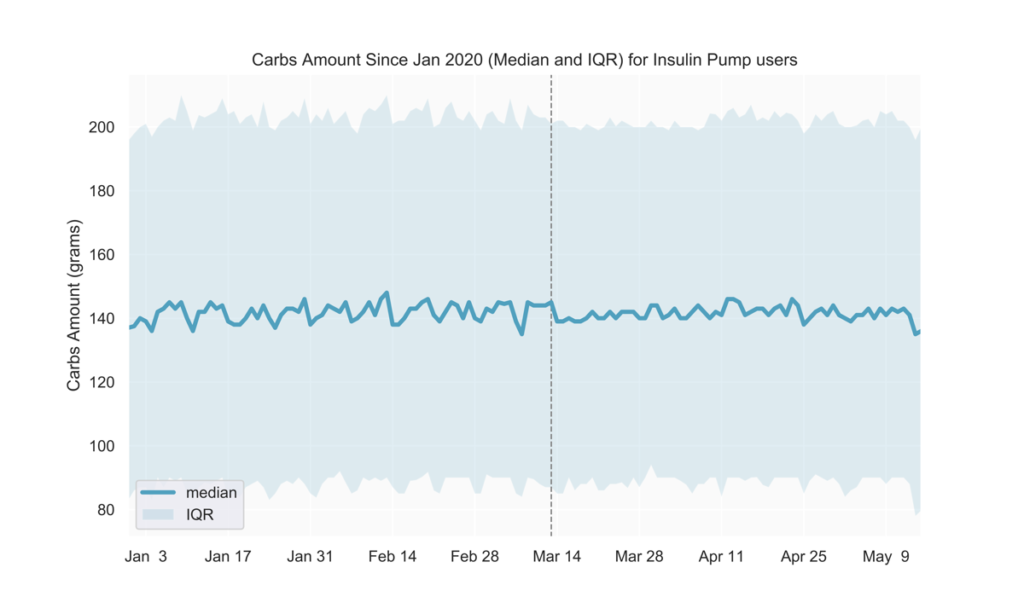
No change in insulin amounts
We observed no discernible changes in the total amount of daily bolus insulin dose among insulin pump users. We will continue to track insulin use to understand if possible disruptions in obtaining prescription drugs will reflect in users’ daily diabetes management. (Fig 6)
Fig 6.
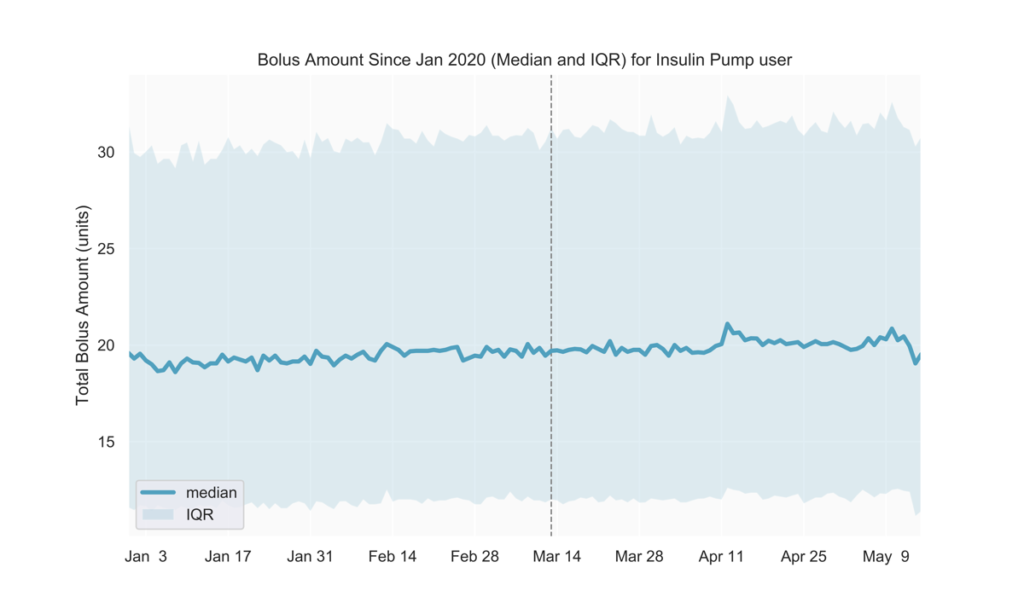
Decreased activity
Glooko users are able to easily track their activity and how it affects their glucose by connecting their preferred activity trackers and apps to the Glooko Mobile app. By analyzing daily steps, we observed a drop in activity post-March 13 as seen in Fig. 7. This was an expected outcome given national shelter-in-place orders in the USA.
Fig 7.
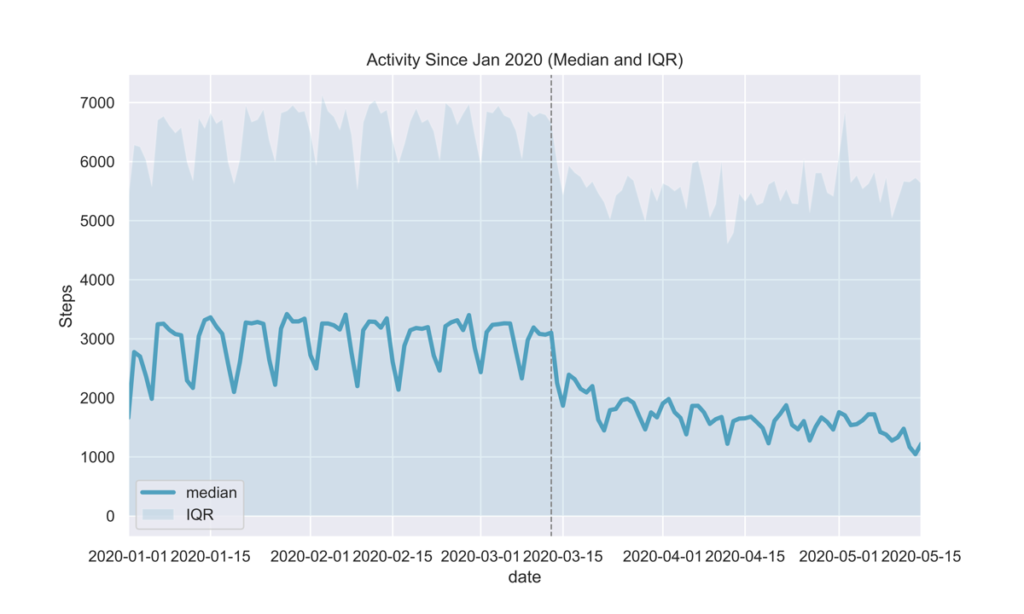
METHOD DATASET
Glucose, insulin, and activity data were sampled from in-clinic and at-home Glooko users in the USA between January and April 2020, and analyzed separately for two main cohorts:
- CGM users: People with diabetes who use a continuous glucose monitoring device,
- non-CGM users: People with diabetes who rely on glucose meters and/or insulin pumps for their diabetes management.
INCLUSION CRITERIA
This analysis included CGM users who had at least 144 CGM readings on any given day, and non-CGM users who had at least 1 glucose reading on any given day.
DEFINITIONS
- Average glucose
- non-CGM users: the average value of glucose readings on a given day in mg/dL
- CGM users: average estimated glucose value on a given day in mg/dL
- Time In Range (TIR), reported only for CGM users: the percentage of estimated glucose values between 70-180mg/dL on a given day
- Rate of Hyperglycemia reported only for CGM users: the number of readings above 180mg/dL on a given day
- Total Bolus Amount reported only for insulin pump users: the total units of insulin (normal and extended) on a given day
- Carbs Amount reported only for insulin pump users: the total amount of carbs that were entered into the insulin pump on a given day
MKT-0213 01